The Impact of Working Capital Management on Corporate Liquidity
Working capital management is recognized as one of the most important managerial tools for improving liquidity and reducing financial risks in companies. Liquidity refers to a company's ability to meet short-term financial obligations without requiring emergency financing. Effective management of working capital, including the optimization of inventory, receivables, and short-term liabilities, can significantly enhance a company's liquidity. This article examines the impact of working capital management on corporate liquidity. This research is a narrative review using a descriptive analysis method. Data was collected through a thorough review and analysis of scientific articles and previous studies on working capital management and liquidity. Sources were retrieved from reputable databases and selected based on relevance, academic quality, and recent publication. The findings show that optimized working capital management, especially in managing inventory and receivables, can improve a company’s liquidity. Furthermore, the use of modern technologies in financial management helps reduce costs and increase liquidity. Additionally, challenges such as financial constraints and short-term debt management can negatively impact liquidity. Working capital management plays a critical role in enhancing corporate liquidity. Companies that employ modern methods for effective working capital management can mitigate financial risks and increase their financial stability.
The Free Cash Flow Theory and Its Implications for Dividend Policy Decisions
Free cash flow plays a crucial role in corporate financial decision-making, particularly in dividend policy. Its significance lies in its direct impact on liquidity, investment opportunities, and overall corporate performance. This study aims to examine the impact of free cash flow on corporate dividend policy and analyze how it influences management decisions regarding dividend payments to shareholders. This study employed a narrative review method, drawing from previous scholarly articles and research related to free cash flow and dividend policy. Data were collected from credible academic sources and analyzed descriptively. The findings revealed that companies with high free cash flow tend to distribute more dividends to shareholders due to having sufficient liquidity for both internal investments and dividend payments. Additionally, in companies with limited investment opportunities, excess free cash flow is more likely directed towards dividends. On the other hand, companies with lower free cash flow prefer to retain cash for potential investments. Dividend policy also acts as a mechanism to control managerial behavior and reduce agency problems. Free cash flow has a significant influence on dividend policy. Financial managers should consider liquidity status, investment opportunities, and strategic goals when making decisions about dividend payments. Furthermore, paying dividends can enhance investor trust and increase a company's stock value.
Applying Deep Learning to Detect Revenue Recognition Fraud
Financial fraud, especially in revenue recognition, poses significant challenges to modern financial systems, directly impacting transparency and public trust in financial markets. Traditional fraud detection methods have limited effectiveness in analyzing complex and large datasets. Deep learning, as an advanced artificial intelligence technology, demonstrates strong capabilities in detecting hidden and unusual patterns in financial data. This study aims to explore the application of deep learning in detecting revenue recognition fraud and compare its effectiveness with traditional methods. This descriptive-analytical study reviews the role of deep learning in detecting financial fraud. Data used include scientific articles and previous studies related to deep learning in the financial domain. Data analysis was conducted using qualitative methods, comparing the performance of deep learning with traditional techniques. Deep learning has shown superior performance in detecting complex and sophisticated fraud compared to traditional methods. It has achieved higher accuracy in identifying suspicious transactions and reducing false alarms. Additionally, deep learning has the capability to predict future fraud and uncover hidden fraud patterns, and it outperforms traditional methods in analyzing multidimensional financial data. Deep learning is recognized as an effective tool for detecting financial fraud and can enhance financial transparency by improving accuracy and efficiency. However, challenges such as the need for large datasets and ethical concerns related to AI use in this field must be addressed.
The Effects of Dividend Policy on Stock Price Volatility: A Review Study
Dividend policies are among the most critical financial decisions for companies, significantly influencing stock price volatility. These policies play a vital role in shaping investor behavior and assessing the financial stability of companies. Stock price volatility, a key factor in investment decisions, is affected by various elements, including dividend policies. This study aims to examine the impact of dividend policies on stock price volatility across different markets. This study is a narrative review utilizing descriptive analysis. Relevant academic sources were collected from various databases, focusing on empirical and theoretical studies that analyze the impact of dividend policies on stock price volatility. Studies from both emerging and developed markets were reviewed to provide a comprehensive understanding of the effects of dividend policies. The results show that companies with stable dividend policies tend to experience lower stock price volatility. Conversely, companies with inconsistent or irregular dividend policies generally witness higher volatility. These effects vary depending on the market type, with emerging markets showing a stronger correlation between dividend policies and stock price volatility due to lower information transparency. Stable dividend policies can help reduce stock price volatility and enhance investor confidence. Additionally, factors such as profitability, liquidity, and macroeconomic conditions also play a crucial role in shaping these impacts.
Analyzing Corporate Bankruptcy Prediction Models Using Machine Learning
Corporate bankruptcy prediction is a critical and complex topic in finance and management that helps prevent financial crises and aids in better decision-making regarding financial risk management. In this regard, the use of machine learning algorithms, due to their ability to process complex data and provide accurate predictions, has emerged as a modern tool in this domain. This study aims to review and compare different machine learning models for corporate bankruptcy prediction and analyze the challenges and limitations of these models. This research follows a systematic literature review method, analyzing relevant scientific articles on the use of machine learning in bankruptcy prediction. Various models, including decision trees, random forests, artificial neural networks, and support vector machines, are examined, with their accuracy and performance evaluated based on empirical data. The results indicate that more complex models, such as neural networks and boosting algorithms, perform better than simpler models like decision trees when dealing with complex and multidimensional data. However, these advanced models face challenges such as interpretability issues, the need for large datasets, and complex parameter tuning. Machine learning techniques can provide high accuracy in predicting corporate bankruptcy, but improvements are needed in addressing challenges like data imbalance, enhancing data quality, and developing more interpretable models.
The Impact of IFRS Adoption on Financial Transparency
International Financial Reporting Standards (IFRS) have been introduced as a crucial tool for enhancing financial transparency and improving the quality of financial reports globally. The adoption of these standards has had positive effects on financial transparency and investor confidence in various countries. This study aims to examine the impact of IFRS adoption on financial transparency and analyze the challenges and benefits associated with its implementation in different financial systems. This research employs a descriptive-analytical method, drawing on a review of previous studies and research on the effects of IFRS. The data includes credible scientific articles and financial reports from various countries, examining the changes in financial transparency before and after IFRS adoption. The findings indicate that IFRS adoption has had positive effects on increasing financial transparency, improving the quality of financial information, reducing information asymmetry, and strengthening investor confidence. Additionally, the comparability of financial reports at the global level has improved. However, challenges such as high costs and the need for adequate infrastructure for implementation exist. IFRS adoption is recognized as an effective tool for improving financial transparency and enhancing trust in financial markets. Although its implementation comes with challenges, the long-term benefits of IFRS, such as improved financial performance and attracting more investments, are evident. Providing proper infrastructure and training personnel can facilitate the implementation of these standards.
Predicting Mergers and Acquisitions Success with Machine Learning
Mergers and acquisitions (M&As) are critical strategies for organizational growth and expansion in modern business environments. However, predicting the success or failure of these processes is a complex challenge due to the multiple factors involved. Traditional models often fall short in accurately forecasting outcomes because they cannot analyze multidimensional and complex data. Machine learning has emerged as an advanced analytical tool that can handle large datasets and improve the accuracy of predictions. To explore the application of machine learning in predicting the success of M&As and analyze its advantages and challenges. This study is a narrative review that draws upon credible academic sources and databases to collect relevant information. Various machine learning models, such as neural networks, support vector machines, and decision trees, are examined and compared with traditional prediction models. The collected data from previous studies and relevant research were comprehensively analyzed. Machine learning demonstrates the ability to analyze multidimensional data and uncover hidden patterns that traditional methods overlook. This analytical tool significantly improves the accuracy of M&A success predictions and helps reduce risks associated with these processes. However, challenges such as data quality and the need for sufficient resources remain prevalent. Machine learning has proven to be an effective tool in predicting the success of M&As by providing more precise analyses of complex data. Implementing this tool, along with improving data quality and proper post-merger management, can lead to greater success in M&A processes.
The Future of Accounting: How AI is Reshaping the Role of Accountants in Financial Reporting
With rapid advancements in technology, artificial intelligence (AI) has emerged as a key driver of change in accounting and financial reporting. This technology, through automation of financial processes and reduction of human errors, has fundamentally transformed the roles of accountants and the way financial activities are conducted. The aim of this article is to examine the impact of AI on the evolving roles of accountants and financial reporting processes. This descriptive review study analyzes past research to explore the effects of AI on accounting and the associated challenges and opportunities. Data for this study were collected and analyzed from reputable scientific databases. The findings indicate that AI, by reducing repetitive tasks, has enabled accountants to focus on advanced analytics and strategic financial advisory roles. While ethical and legal challenges remain, AI presents emerging opportunities for improving financial processes and elevating accountants' roles. The adoption of AI in accounting has led to fundamental changes in the profession, requiring accountants to adapt by acquiring new skills. Organizations must also invest in the necessary infrastructure to implement this technology and prioritize continuous staff training. Future research should focus on addressing the legal and ethical challenges related to AI in accounting.
About the Journal
Owner: Research Institute for the Development of Knowledge and Research
Publisher: Maher International Publication
Phone: +982166859278
Address: No. 25, 37th Street, After the Third Roundabout, Tehran Pars, Tehran.
Accounting, Finance and Computational Intelligence is a prestigious open-access journal dedicated to advancing scholarly research at the intersection of accounting, finance, and computational intelligence. The journal provides a dynamic platform for academic researchers, industry professionals, and policy-makers to share cutting-edge developments, empirical studies, theoretical advancements, and applications of computational tools in solving complex problems in accounting and finance. Our commitment to fostering innovation is reflected in the journal's diverse scope, which encourages interdisciplinary research that bridges gaps between finance, accounting practices, and computational intelligence.
We believe that the future of accounting and finance lies in the seamless integration of artificial intelligence (AI), machine learning (ML), and other computational methodologies to enhance the accuracy, efficiency, and predictive power of financial models and decision-making processes. The journal invites submissions that contribute to theoretical advancements, provide practical insights, or present case studies that demonstrate the power of computational intelligence in reshaping the financial landscape.
Current Issue
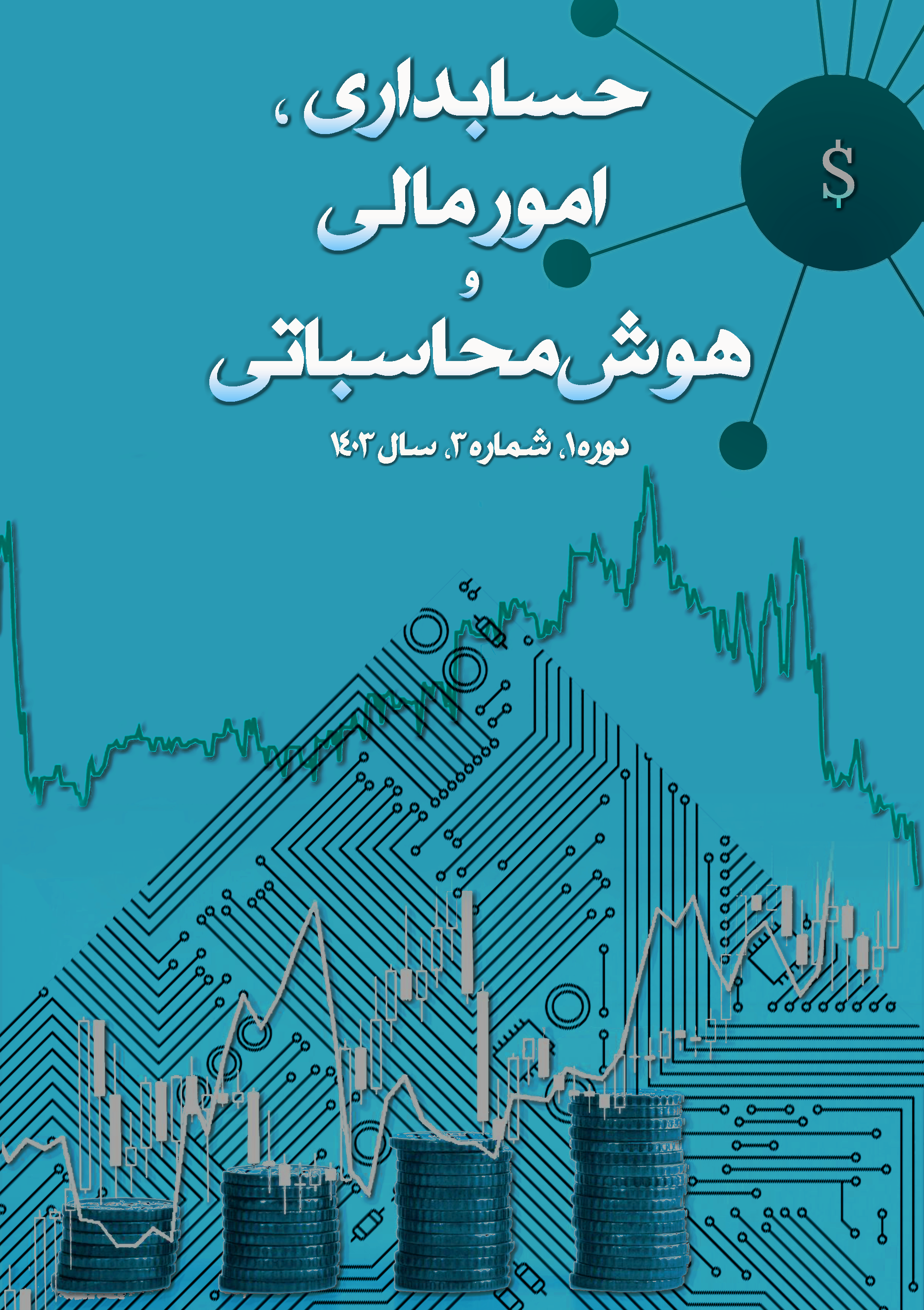
Articles
-
Analyzing Corporate Bankruptcy Prediction Models Using Machine Learning
Mohammad Rezaei ; Ali Ahmadi *13-24 -
The Free Cash Flow Theory and Its Implications for Dividend Policy Decisions
Kaveh Mirzaei ; Niloofar Salehi * ; Ali Abdulrazzaq52-65 -
The Impact of Working Capital Management on Corporate Liquidity
Seyed Ali Tavassoli Yazdi ; Sara Khademi *66-77