About the Journal
Owner: Research Institute for the Development of Knowledge and Research
Publisher: Maher International Publication
Phone: +982166859278
Address: No. 25, 37th Street, After the Third Roundabout, Tehran Pars, Tehran.
Accounting, Finance and Computational Intelligence is a prestigious open-access journal dedicated to advancing scholarly research at the intersection of accounting, finance, and computational intelligence. The journal provides a dynamic platform for academic researchers, industry professionals, and policy-makers to share cutting-edge developments, empirical studies, theoretical advancements, and applications of computational tools in solving complex problems in accounting and finance. Our commitment to fostering innovation is reflected in the journal's diverse scope, which encourages interdisciplinary research that bridges gaps between finance, accounting practices, and computational intelligence.
We believe that the future of accounting and finance lies in the seamless integration of artificial intelligence (AI), machine learning (ML), and other computational methodologies to enhance the accuracy, efficiency, and predictive power of financial models and decision-making processes. The journal invites submissions that contribute to theoretical advancements, provide practical insights, or present case studies that demonstrate the power of computational intelligence in reshaping the financial landscape.
Current Issue
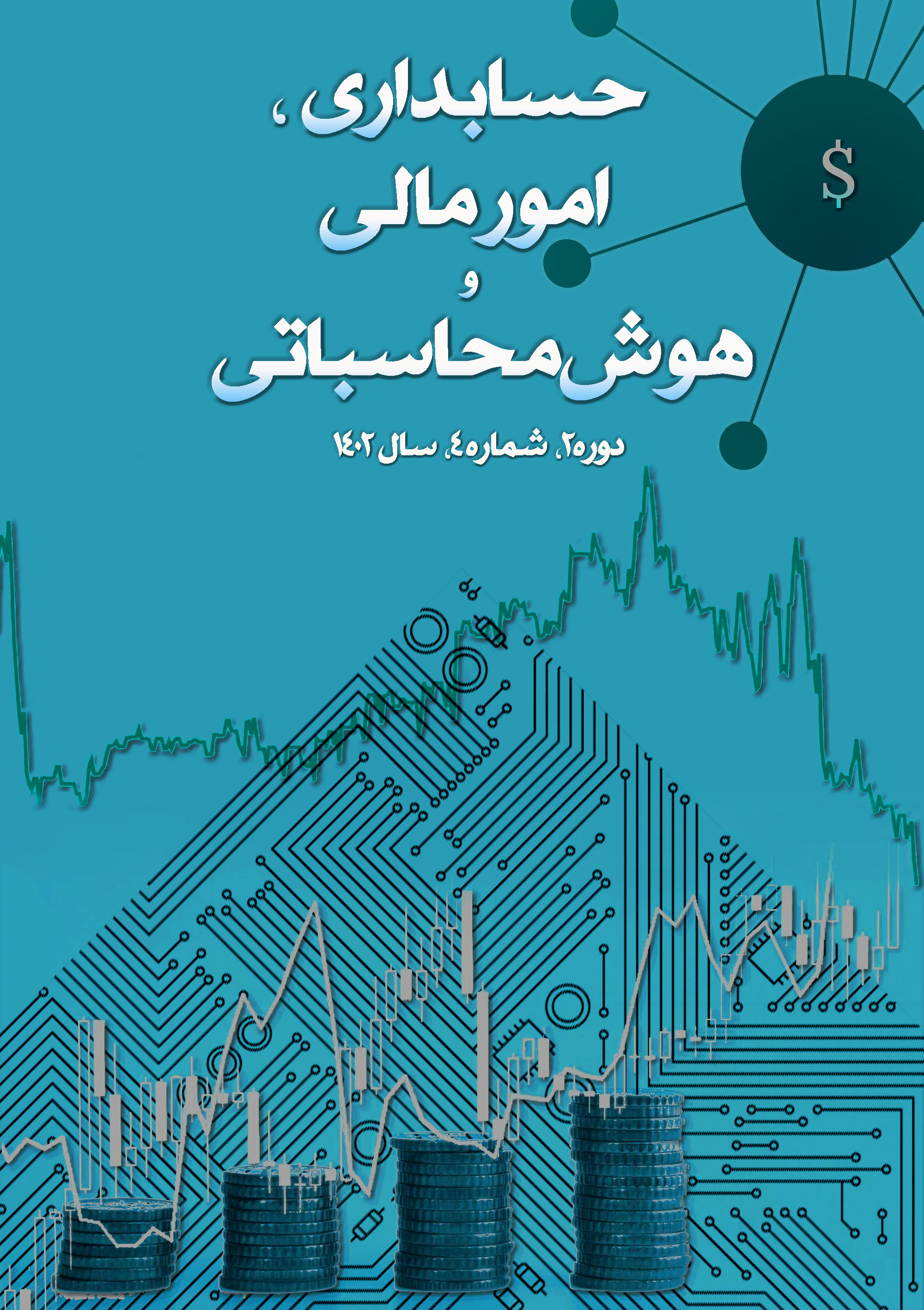